Future-Proofing Retail: Adapting to change with edge to cloud computing
10th July 2024
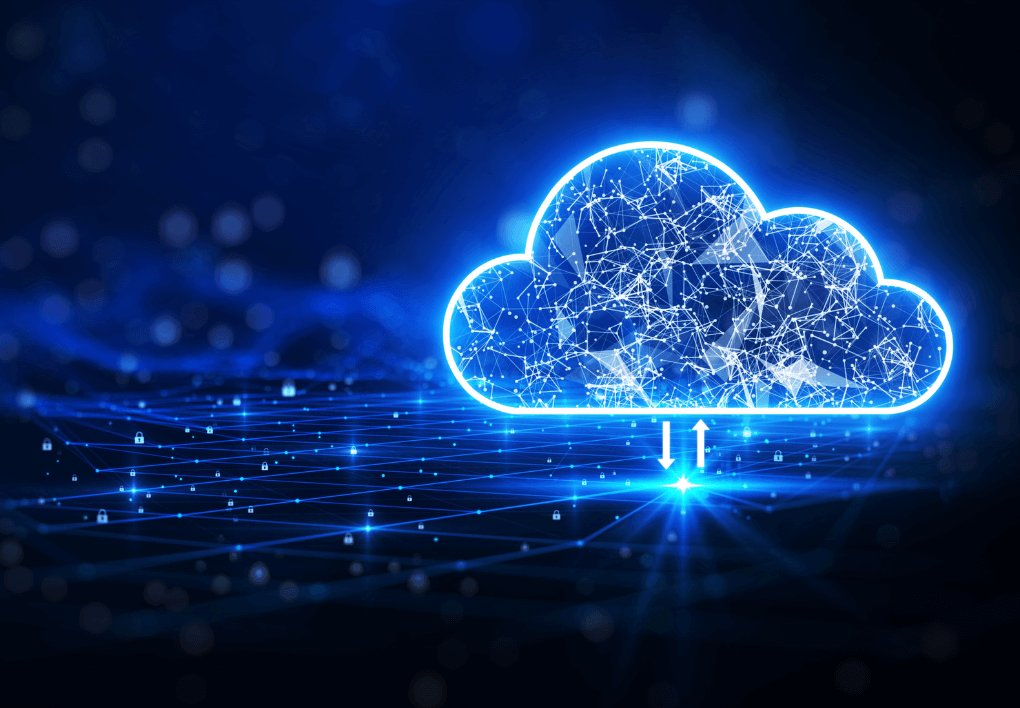
What if you could anticipate the needs of customers and employees in-store, streamline experiences and deliver a wealth of efficiencies? This is retail powered by edge-to-cloud computing, an approach that combines the strengths of local processing in devices (edge) with centralised computing power (cloud). Just as our brains rely on senses and a central processor to make sense of the world, edge-to-cloud connects devices (senses) with the cloud (brain) to create a smarter, more responsive retail.
This blog explores how edge-to-cloud computing is transforming retail, using the self-checkout as an example and explaining its core concepts through a brain-body analogy.
Edge-to-cloud computing: A technical definition
Edge-to-cloud computing refers to a distributed computing architecture that combines the capabilities of edge devices (such as security cameras) with cloud infrastructure to process and analyse data. In this architecture, data is collected, processed, and analysed both at the edge (i.e. closer to where it is produced and consumed) and in the cloud (i.e., in centralised data centres).
Edge-to-cloud computing: Without the technical jargon
Imagine your brain as a central processing unit (CPU) of a computer. It receives information from your senses (like edge devices) and analyses it. Edge-to-cloud computing behaves in the same way.
For example, imagine your hand automatically pulling away from a hot stove – that’s edge processing in action! Edge devices collect data and handle basic tasks on their own, reducing latency (think: reaction time).
They can also connect to the cloud for complex analysis, like storing data or sending updates (remembering the hot stove for next time). These connected devices, known as the Internet of Things (IoT), power “smart” technology by enabling automation, efficiency gains, and data-driven innovation.
Imagine edge devices as ‘your’ senses
Just as we understand the world through our senses, edge devices make sense of the world with sensors. For example, supermarket self-checkouts utilise several sensors including:
- Cameras (eyes): Just like eyes capture visual data, environmental cameras take pictures or videos of their surroundings.
- Barcode scanners (touch): Barcode scanners, like our sense of touch, gather specific information about objects close by. They read unique codes to identify and track items.
- Weighing scales (pressure sensors): Similar to how we estimate the weight of objects by holding them, weigh scales measure weight or force applied to them.
This sensory data is the raw input, just like the information our senses transmit to our brain. When we extract value from this data, by sending the information on to our brain, we can then learn from it.
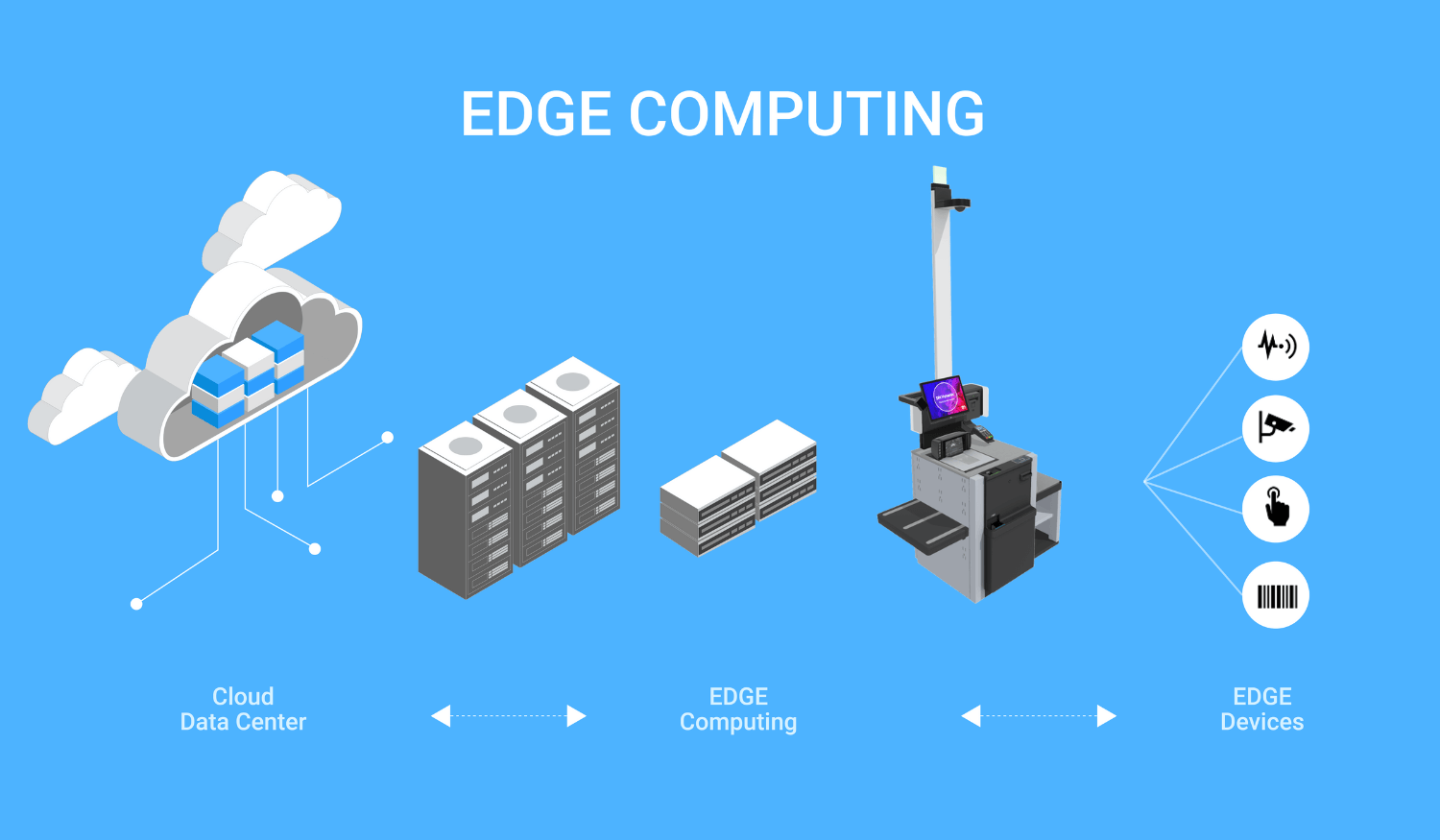
Local processing is akin to the nervous system
While edge devices excel at basic tasks like object detection, the real power comes from their collaboration with the cloud:
- Edge Processing (central nervous system): Just as your nervous system reacts instantly, triggering your hand to pull away from a hot stove, edge devices can process data locally and take immediate action. This is similar to how your nervous system reacts instantly without needing your brain’s full awareness.
- Cloud computing (brain): The cloud acts as the compute resource, like your brain. It receives all the data from edge devices and analyses it for deeper insights, just as your brain learns from experiences. This allows the system to make smarter decisions – for example, in the future you might check the stove before touching it, thanks to the “hot stove” experience processed by your brain.
In this way, cloud computing acts as the brain, taking the data from the edge devices, analysing it, and storing information for future reference.
Machine learning: making sense of the data
Our brains excel at storing data and recognizing familiar patterns (like a hot stove!), but struggle to manage vast amounts of new information.
Think of Machine Learning as a brain booster for data. Machine learning algorithms analyse data from edge devices, uncovering patterns, trends, and anomalies beyond simple recognition.
Take self-checkout weigh scales. They block discrepancies but lack the nuance to understand the cause. This is where machine learning steps in.
Actionable data: your decisions and actions
The rich data from edge devices reveals patterns and anomalies, allowing for smarter actions. Think of it like your brain interpreting sensory data. This actionable data, or metadata, in the case of a self-checkout triggers responses like:
- Alerts for mismatched barcodes or forgotten scans
- Security notifications for activity of interest
With this intelligence, the system can react with nuance. For example, AI-powered scales wouldn’t block with every discrepancy, but only those truly requiring intervention.
The Loop: Continuous learning
Like our brains constantly learning, data from edge devices refines the cloud’s machine learning, leading to even smarter insights.
This continuous loop, akin to a distributed nervous system, gathers information, processes it centrally, and fuels intelligent real-world actions. Edge-to-cloud goes beyond simply connecting devices; it creates a smarter, responsive, and user-centric environment.
How does edge-to-cloud help retail? Self-checkout example.
Imagine self-checkout that’s as fast as a cashier, but without the wait. Edge-to-cloud computing makes that possible.
Even more exciting is the integration of machine learning with edge devices. The vast amount of data collected at the edge can also be sent to the cloud for analysis by powerful machine learning algorithms. These algorithms can then be used to train and improve the self-checkout system itself.
And as more real-time analysis of actions taken by shoppers at self-checkout become the norm, with the desire to reduce employee intervention, there’s a need for increased compute or processing power together with a lower tolerance for time delay or latency between ‘event taken by shopper’ and ‘corrective action’, such as SCO-generated nudges for item mis-scans. The logical approach is edge-to-cloud computing to reduce the latency between where the information is collected, processed and consumed.
This aligns with Gartner’s prediction that by 2026, at least 50% of edge computing deployments will involve machine learning, compared with only 5% in 2022. By leveraging machine learning at the edge, self-checkouts will become even smarter, faster, and more secure.
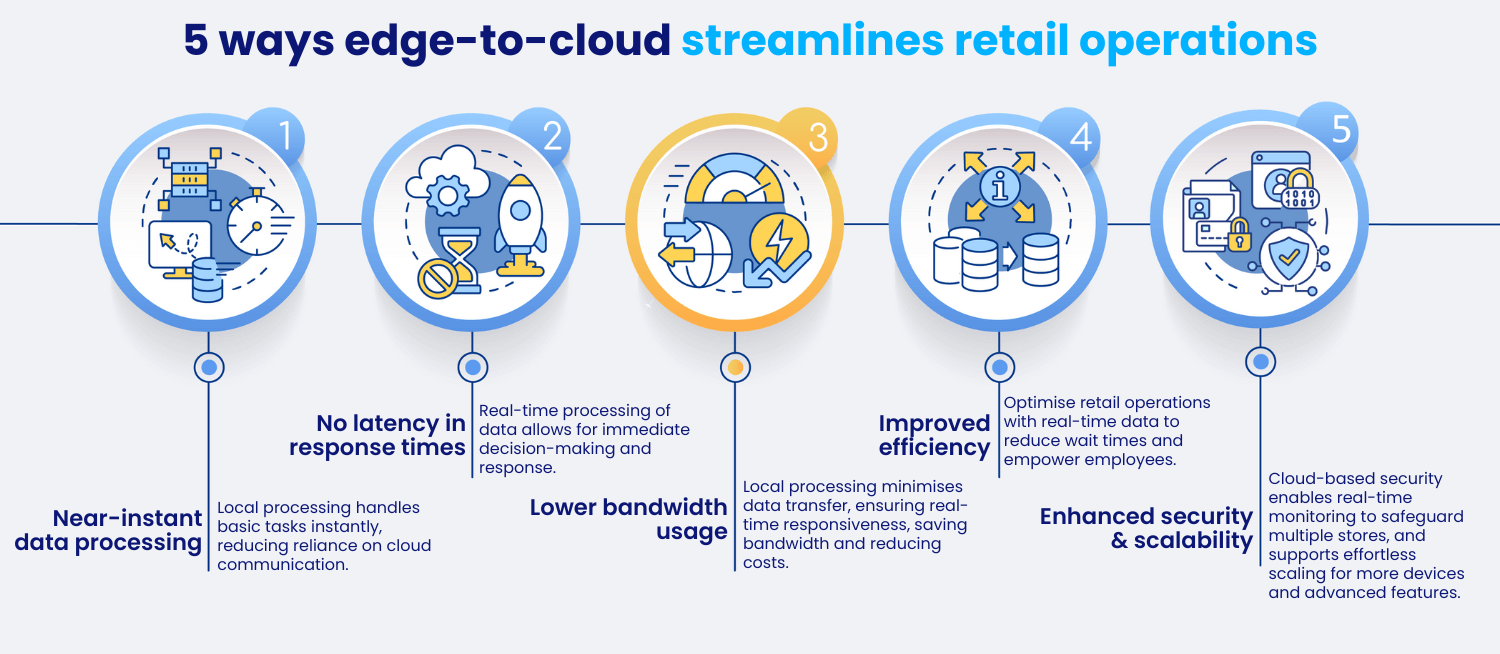
5 ways edge-to-cloud streamlines retail operations
By combining edge and cloud capabilities, self-checkouts achieve a level of performance previously unseen:
- Reduced network traffic: by only sending data when necessary, the SCO minimises the amount of data flowing to the cloud, saving bandwidth and reducing costs
- Faster response times: Local processing at the self-checkout handles basic tasks instantly, reducing reliance on cloud communication and minimizing delays caused by network latency. This translates to smoother and faster transaction processing.
- Enhanced functionality: the cloud can perform complex analysis and automation based on the data received from the self-checkout, such as identifying suspicious activity or updating software. Meanwhile, the edge processing allows for a more immediate user experience by enabling a smooth and quick checkout process without needing to wait for cloud authorisation for every action.
- Improved efficiency: By utilising the cloud functionality as needed, the SCO is armed with more information and is able to manage more complex scenarios – such as nudging customers to correct mistakes rather than blocking the checkout and calling an assistant to intervene. This frees up staff to focus on other tasks, or assist customers who need help. Overall this reduces wait times, and improves checkout efficiency for both customers and employees.
- Security and scalability: Real-time monitoring and anomaly detection improve security, while the system easily scales to accommodate more devices and advanced functionalities.
The intelligent edge for retail
Just as edge-to-cloud computing is revolutionising efficiency and loss prevention at self-checkouts it’s also paving the way for a more connected and efficient retail experience. This distributed computing future will see edge devices handle more complex tasks, reducing latency and boosting efficiency.
The result is a smoother journey for customers and the ability to unlock a wealth of data-driven insights for better business decisions.